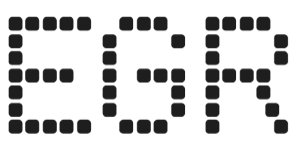
Promoted feature: The future is modelled
Rory Donegan and Dave O’Riordan outline the shift to more in-depth tools for modelling marketing performance

Measuring digital marketing performance using attribution has been the norm in the Real Money Gaming (RMG) industry for the last 10 or more years. It’s not the only tool available, but it’s certainly the one with the quickest and most tactical insights, giving almost real-time KPIs. But that’s changing. Digital marketers are adapting and expanding their horizons to include a more modelled approach to measuring marketing performance.
Why now and what’s changing?
For all the user-level, tactical insights that attribution provides, it still has limitations: attribution doesn’t account for all marketing channels (e.g. TV, out of home (OOH), print etc. and it doesn’t provide incremental results. All conversions are attributed to an activity, even if they would have happened anyway.
These limitations have always existed and coupled with the evolving signals landscape, it means marketers might need to re-evaluate how they’re assessing performance and ask if the way they’ve always done it still makes the most sense.
We’re at a tipping point where leveraging a privacy-centric solution that also addresses attributions limitations might start to make more sense. It affords digital marketers an opportunity to measure all their activity on a level-playing field and protect their budgets.
Marketing Mix Modelling– an old tool for a modelled future
Marketing Mix Modelling (MMM) is a tool that has been around for more than 50 years. Some form of it is likely already in use in most organisations in the RMG industry. If attribution is building a picture of performance from the bottom up (user-level), MMM is using statistical modelling to give a top-down (aggregate) view of how advertising is performing for both active customers and acquisition.
What does MMM do well and how is it currently used?
MMM takes a bird’s-eye view of all activity that could potentially impact sales. This means that traditional and non-traditional channels are accounted for in the same model, unlike attribution which only accounts for digital channels. It makes sure that credit for the same conversion isn’t given to multiple channels across different tools, so there’s one source of truth. TV, out of home and print, for example, all appear alongside digital publishers such as display and search.
This gives marketers a view of the incremental performance of their activity, taking into account non-marketing drivers like competition, economic factors and seasonality. In accounting for both marketing and non-marketing, it can clearly identify the incremental results versus those that would have happened anyway. This helps get to a more accurate return on investment (ROI) for each activity.
MMM results can be leveraged in a number of ways. Primarily a comparison of ROIs across all marketing channels makes for simple application to budget allocation. The model can be used to run simulations on future marketing plans, forecast best- and worst-case scenarios using different budgets and non-marketing trends. It can also be used to inform in-channel strategy and get tactical insights (more on enabling this later).
But, like anything, it’s not perfect and isn’t a like-for-like replacement for attribution. It’s undoubtedly a slower form of measurement than those already in use by digital marketers. Models are usually updated quarterly, twice yearly, or even yearly, but that’s changing. Some third-party providers offer monthly updates and business tracking for advertisers. It’s not as granular as advertisers are used to, but data science teams are adapting their methodology to include shorter, deeper models while maintaining robustness. This allows them to leverage the model to inform tactics, and not just budgeting or broad strategy.
How can we make sure that all channels show up?
One criticism levelled at MMM is that bigger investments ‘show up’ better. To a certain extent, this is true, and there are two reasons why:
- Usually, the bigger the investment, the easier it is to ‘pick up’ in a model. This is a half-truth. Investment level definitely matters, but so does performance and impact on conversions. A combination of both is usually required to be able to capture accurately in a model. As a rule of thumb, your investment on a channel should be a minimum of 2% if you’d like it measured.
- Traditional media has always been measured this way. These teams are involved in the MMM planning conversation, leveraging the technique from the outset to answer questions they have. These channels therefore garner the most attention and focus of modellers.
For big-ticket investments, like TV, measurement in MMM can be relatively simple. The modeller gets weekly TV GRPs, knows what campaigns they are against and can split them in the model delivering a different result for each.
For more tactical channels (e.g. social and display) where share of wallet might be lower, measuring the impact can be harder. In this case, advertisers can revert back to using attribution results which aren’t incremental. There are ways that you, as marketers, can ensure your channels are more fairly represented in these bird’s-eye view models.
Ensure you’re capturing all activity
If the model doesn’t have an input, it won’t have an output. Make sure the modelling team has access to all the activity you’ve been running.
Get access to the best possible data source to be used in the model
Most models are run at a weekly level, often by region, to align with TV data from Nielsen or IRI. So, you should work towards getting data to your modelling team or third-party provider to this level at the very least. Monthly spend figures from a media plan won’t be sufficient. All digital publishers should be able to source data at a level that will improve the fairness of measurement, so please ask the question.
Provide context to the modelling team on the activity that was run
Group activities in a logical way, which can be leveraged when it comes to decision making.
The model won’t know that brand activity might have a longer-term impact than DR activity and so ensure this context is provided.
Similarly, make sure grouping by how the activity was bought is considered. If the question you’ve answered isn’t replicable in planning and execution, it’s a nice-to-know.
Get involved! Discuss strategy and tactics with your team before making recommendations. Don’t assume they’ll have the same questions as you, or that they’ll interpret marketing activity in the same way.
If you’ve got a burning question keeping you up at night, ask it! The worst you’ll get is a no. At best, you can get strategic guidance on marketing investments that can increase performance.
As we near the end of a particularly unusual year, budgeting decisions for 2021 will be front and centre. Now is the time to reflect on where each GBP has been spent in the previous 12 months. Explore the channels, strategies and tactics that worked or didn’t work, and decide where and how to effectively allocate investment for the next year. I encourage all of you to try being involved in this conversation, and leverage MMM to give guidance now and in the future. As you do, this type of modelled solution will be your best chance at equal measurement for all.
To find out more about our products and solutions, please contact us at rmg@fb.com.
Check out fb.gg/marketers for all the latest gaming news, advertising tips, best practices and case studies to meet your business goals.

Rory Donegan (left) and Dave O’Riordan (right)
Rory Donegan joined Facebook’s EMEA Gaming team as a marketing science partner in 2019, to promote better measurement across the industry. He spent the previous seven years working with an analytics consultancy, helping advertisers across EMEA measure and improve their marketing strategy.
Based in Dublin, Dave O’Riordan is a client solutions manager on Facebook’s Real Money Gaming Team. Prior to joining Facebook, he was part of Paddy Power Betfair’s online media team where he helped execute numerous campaigns, including those for Cheltenham, Euro 2016 and World Cup 2018.